On a baking Wednesday two weeks ago, two teams of journalists, YouTubers, and bloggers duked it out on Sutton United’s unforgiving 3G pitch (just ask my legs) while Opta, collectors and distributors of sports data, observed and recorded the match action as they would a proper game.
This was not a proper game. You don’t haul over twenty men and women of questionable fitness out of a collection of offices and get a proper game. But what you do get, having taken part in it, is a very real sense of what it’s like to play football under the stats microscope, and just how much football data can and does shape the modern game we watch and read and write about.
I am a goalkeeper, which obviously made my participation a little different. No easy sideways balls for me to maintain a decent passing percentage. I was to be measured on shots on target saved, some measure of distribution (though that’s never been a strong point), and crosses claimed or dropped or, as we shall see, elbowed into my own net.
The post-match analysis was, in part, powered by Opta’s Expected Goals model and, indeed, much of the event seemed geared to persuading us that xG is ‘good’ and possibly even ‘the future’.
A moment of disclaimer, here: I am a fully paid up member of the stats club, having once even gate-crashed the drinks event that follows the Opta annual conference, and I’ve used stats working for several companies, including the one that powers the Premier League’s own matchday blogs and social feeds. So I am, effectively, a customer as much as a consumer of football data, and Opta didn’t need to convert me. Nonetheless, I learned some stuff from my involvement in the match that surprised me.
So, what is Expected Goals? Essentially, it’s a probabilistic measurement of the likelihood of a shot from a recorded location going in, based on all the data for shots from that location previously gathered. Known as xG, it’s actually very handy, and I was an advocate until I let in a Michael Cox effort with an xG of 0.03, or 3%, at which point expected goals became an object of loathing. (For what it’s worth, there were five players between me and the Cox boot, so I only saw the shot very late, by which time my leaden body had insufficient opportunity to heave itself downwards.)
Opta have also developed an xGOT, nothing to do with that show, which is expected goals on target. xGOT varies from xG in that xG records simply the likelihood of a shot going in based on location and angle in relation to the goal, whereas xGOT then factors in that the shot is from a smaller sample of such shots that have hit the target. xGOT is thus higher than xG.
For the efforts I faced, the xG was a measly 3.71, but the xGOT was 5.32 – take away my aforementioned own goal, where I had to come for a cross and managed to deflect it in, and the discrepancy between the seven goals conceded and the 5.32 xGOT means I let in maybe one or two more than I should have. I put that down to two factors: one, the tangible quality of the opposition, and secondly my intangible growing sense of exhaustion and despair.
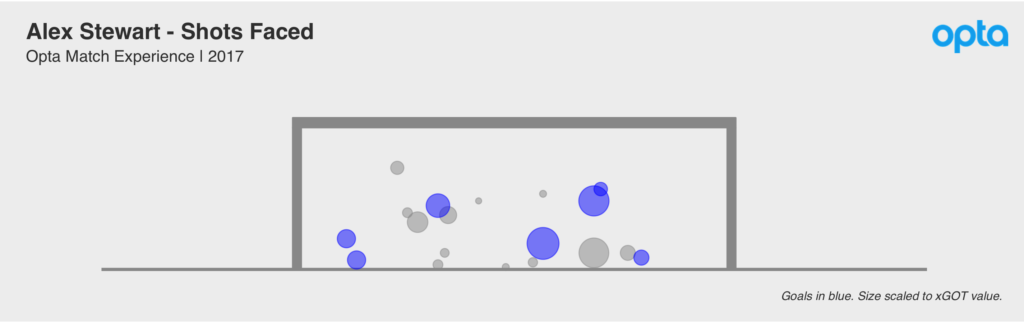
Goals are in blue. Size of the circle shows the likelihood of that shot going in based on its xGOT.
Where I stood out, though, was in saves made. I faced 19 shots on target (not counting my O.G.) and made 12 saves, a 63.2% save percentage, which puts me between Maarten Stekelenburg (63.6%) and Lukasz Fabianski (62.5%) for Premier League stoppers.
Twelve saves in one match is a lot, though: in last season’s Premier League, only Vito Mannone made more in a single game – 13 against Liverpool. The second most saves in one match was 11, recorded twice by Tom Heaton against Manchester United and Southampton, and once by Jordan Pickford against Arsenal. Yes, I had to do more than Sunderland’s goalkeeper had to do against Arsenal. Let that sink in, my friends.
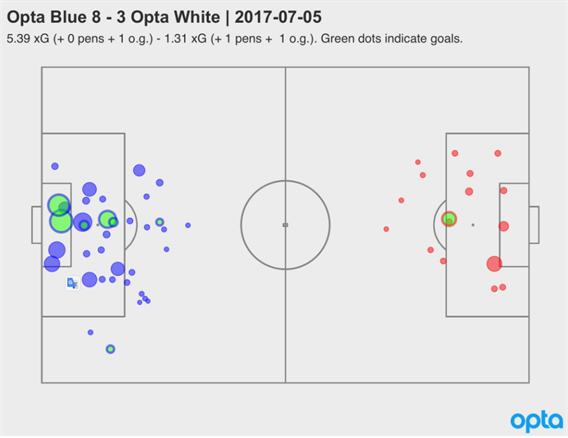
Match xG table: Size of circle indicates a greater likelihood of that shot going in.
What was most interesting, though, was the psychological impact all this measurement had on me. At half time, trailing 5-0 or 5-1 (as a keeper, I am not much fussed about how many we’ve scored), I was told I had made nine saves and faced 14 shots on target (“Yes, I can do maths”, was my very grumpy retort, for which I now, tardily, apologise to the gaffer).
Taking to the pitch again, these nine saves were huge in my mind. I started to think about reaching double figures, then about making more saves than Heaton against Southampton, which I remembered as a disgruntled Saints fan (I had forgotten Mannone and, in any event, I failed to match him).
But for someone like me, obsessive and numerate to a degree, knowing my performance and wanting to better it became a reason in itself for that performance. I didn’t want my team to win, not that it could, quite so much as I wanted to outperform Tim Howard’s 16 meme-worthy saves against Germany.
As it was, my side rallied brilliantly in the second half and I never quite got the chance, but still, it was there, front and centre in my thinking. One more mistake from a centre-back, and I might get another save. It’s a very, very odd way to think about football as a player and I’m sure a reflection of the sort of solipsism that led me to being a ‘keeper in the first instance; I don’t know how many others were thinking of their performance in the same way, although for an outfielder scoring or getting an assist is clearly a measurable aim in itself.
I therefore felt the impact of being measured much more than I thought I would. Clearly playing only one game, and the rest of my football being limited to runs-out with Pickles FC, there is limited developmental use for me in terms of having my performance scrutinised. But it gave me a real insight into what playing under the spotlight must be like, the constant need to measure oneself against what’s happening, and just how hard that must be.
I heard Danny Murphy speak at a recent Opta event about how he was asked, early in his career, to keep a tally of his assists, how often he got into the box, and so on, and how useful that was in terms of seeing when he was doing the right thing and when he was erring. I took my number of saves incredibly seriously, despite the cobbled-together nature of the match, and so I can see, in a parallel world, how this information would have been extremely useful to me as an actual player.
I can also see how Expected Goals, as well as the less opaque metrics collected and/or derived from the game, clearly added a level of understanding to a match I had actually played in.
For someone who didn’t need to be convinced of the use of data in football, I came away from the experience even more certain of its utility. I also learned one, very valuable lesson. Always close down Michael Cox.